RESEARCH HUB
Ellpha team & community are actively gathering all the past and current research available, relevant media, BLOG and sites covering ellpha's domains of interest, and creating a REPOSITORY of people & organisations who care and contribute to AI & diversity.
Please email info@ellpha.com if you want to add any contributions or contacts.
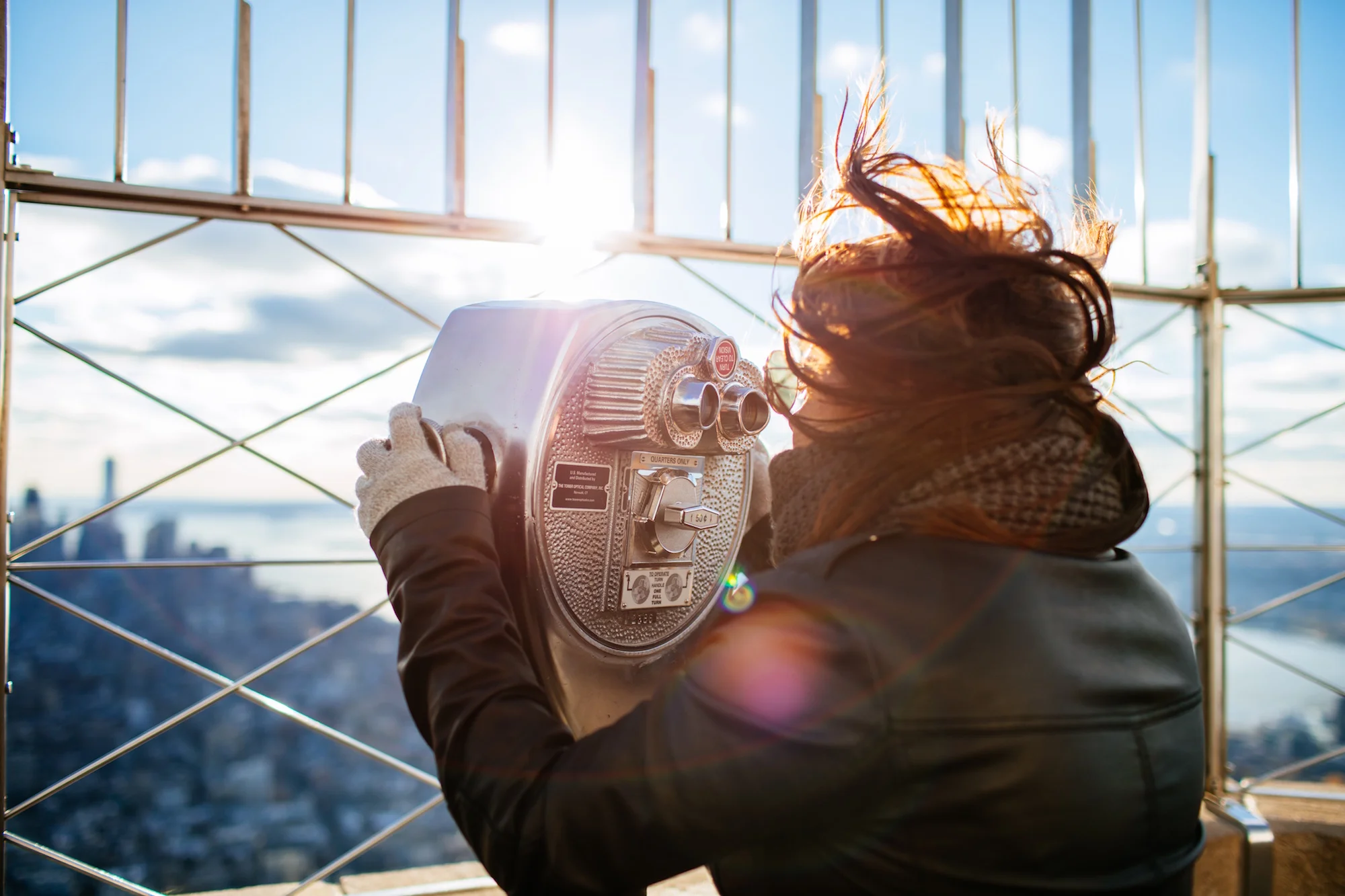
ACADEMIC RESEARCH
Joy Buolamwini joyab@mit.edu MIT Media Lab 75 Amherst St. Cambridge, MA 02139
Timnit Gebru timnit.gebru@microsoft.com Microsoft Research 641 Avenue of the Americas, New York, NY 10011
Recent studies demonstrate that machine learning algorithms can discriminate based on classes like race and gender. In this work, we present an approach to evaluate bias present in automated facial analysis algorithms and datasets with respect to phenotypic subgroups. Using the dermatologist approved Fitzpatrick Skin Type classification system, we characterize the gender and skin type distribution of two facial analysis benchmarks, IJB-A and Adience. We find that these datasets are overwhelmingly composed of lighter-skinned subjects (79.6% for IJB-A and 86.2% for Adience) and introduce a new facial analysis dataset which is balanced by gender and skin type. We evaluate 3 commercial gender classification systems using our dataset and show that darker-skinned females are the most misclassified group (with error rates of up to 34.7%). The maximum error rate for lighter-skinned males is 0.8%. The substantial disparities in the accuracy of classifying darker females, lighter females, darker males, and lighter males in gender classification systems require urgent attention if commercial companies are to build genuinely fair, transparent and accountable facial analysis algorithms.
Keywords: Computer Vision, Algorithmic Audit, Gender Classification
Full Research: http://proceedings.mlr.press/v81/buolamwini18a/buolamwini18a.pdf
Decisions based on algorithmic, machine learning models can be unfair, reproducing biases in historical data used to train them. While computational techniques are emerging to address aspects of these concerns through communities such as discrimination-aware data mining (DADM) and fairness, accountability and transparency machine learning (FATML), their practical implementation faces real-world challenges. For legal, institutional or commercial reasons, organisations might not hold the data on sensitive attributes such as gender, ethnicity, sexuality or disability needed to diagnose and mitigate emergent indirect discrimination-by-proxy, such as redlining. Such organisations might also lack the knowledge and capacity to identify and manage fairness issues that are emergent properties of complex sociotechnical systems. This paper presents and discusses three potential approaches to deal with such knowledge and information deficits in the context of fairer machine learning. Trusted third parties could selectively store data necessary for performing discrimination discovery and incorporating fairness constraints into model-building in a privacy-preserving manner. Collaborative online platforms would allow diverse organisations to record, share and access contextual and experiential knowledge to promote fairness in machine learning systems. Finally, unsupervised learning and pedagogically interpretable algorithms might allow fairness hypotheses to be built for further selective testing and exploration.
In 2017, discussions around gender and media have reached a fever pitch. Following a bruising year at the ballot box, fourth-wave feminism has continued to expand. From the Women’s March to high-profile sexual harassment trials to the increasing number of female protagonists gaining audience recognition in an age of “peak TV,” women are ensuring that their concerns are heard and represented.
We’ve seen movements for gender equality in Hollywood, in Silicon Valley — and even on Madison Avenue. In response to longstanding sexism in advertising, industry leaders such as Madonna Badger are highlighting how objectification of women in advertising can lead to unconscious biases that harm women, girls and society as a whole.
Agencies are creating marquee campaigns to support women and girls. The Always #LikeAGirl campaign, which debuted in 2014, ignited a wave of me-too “femvertising” campaigns: #GirlsCan from Cover Girl, “This Girl Can” from Sport England and the UK’s National Lottery, and a spot from H&M that showcased women in all their diversity, set to “She’s a Lady.” Cannes Lions got in on the act in 2015, introducing the Glass Lion: The Lion for Change, an award to honor ad campaigns that address gender inequality or prejudice.
But beyond the marquee case studies, is the advertising industry making strides toward improving representation of women overall? How do we square the surge in “femvertising” with insights from J. Walter Thompson’s Female Tribes initiative, which found in 2016 that, according to 85% of women, the advertising world needs to catch up with the real world?
Women continue to remain underrepresented in male-dominated fields such as engineering, the natural sciences, and business. Research has identified a range of individual factors such as beliefs and stereotypes that affect these disparities but less is documented around institutional factors that perpetuate gender inequalities within the social structure itself (e.g., public policy or law). These institutional factors can also influence people’s perceptions and attitudes towards women in these fields, as well as other individual factors.
The research presented in this paper demonstrates a model for aiding human-robot companionship based on the principle of 'human' cognitive biases applied to a robot. The aim of this work was to study how cognitive biases can affect human-robot companionship in long-time. In the current paper, we show comparative results of the experiments using five biased algorithms in three different robots such as ERWIN, MyKeepon and MARC.
Recent methods for learning vector space representations of words have succeeded in capturing fine-grained semantic and syntactic regularities using vector arithmetic , but the origin of these regularities has remained opaque. We analyze and make explicit the model properties needed for such regularities to emerge in word vectors. The result is a new global log-bilinear regression model that combines the advantages of the two major model families in the literature: global matrix factorization and local context window methods. Our model efficiently leverages statistical information by training only on the nonzero elements in a word-word co-occurrence matrix, rather than on the entire sparse matrix or on individual context windows in a large corpus. The model produces a vector space with meaningful sub-structure, as evidenced by its performance of 75% on a recent word analogy task. It also outperforms related models on similarity tasks and named entity recognition.
The blind application of machine learning runs the risk of amplifying biases present in data. Such a danger is facing us with word embedding, a popular framework to represent text data as vectors which has been used in many machine learning and natural language processing tasks. We show that even word embeddings trained on Google News articles exhibit female/male gender stereotypes to a disturbing extent. This raises concerns because their widespread use, as we describe, often tends to amplify these biases. Geometrically, gender bias is first shown to be captured by a direction in the word embedding. Second, gender neutral words are shown to be linearly separable from gender definition words in the word embedding. Using these properties, we provide a methodology for modifying an embedding to remove gender stereotypes, such as the association between between the words receptionist and female, while maintaining desired associations such as between the words queen and female. We define metrics to quantify both direct and indirect gender biases in embeddings, and develop algorithms to "debias" the embedding. Using crowd-worker evaluation as well as standard benchmarks, we empirically demonstrate that our algorithms significantly reduce gender bias in embeddings while preserving the its useful properties such as the ability to cluster related concepts and to solve analogy tasks. The resulting embeddings can be used in applications without amplifying gender bias.
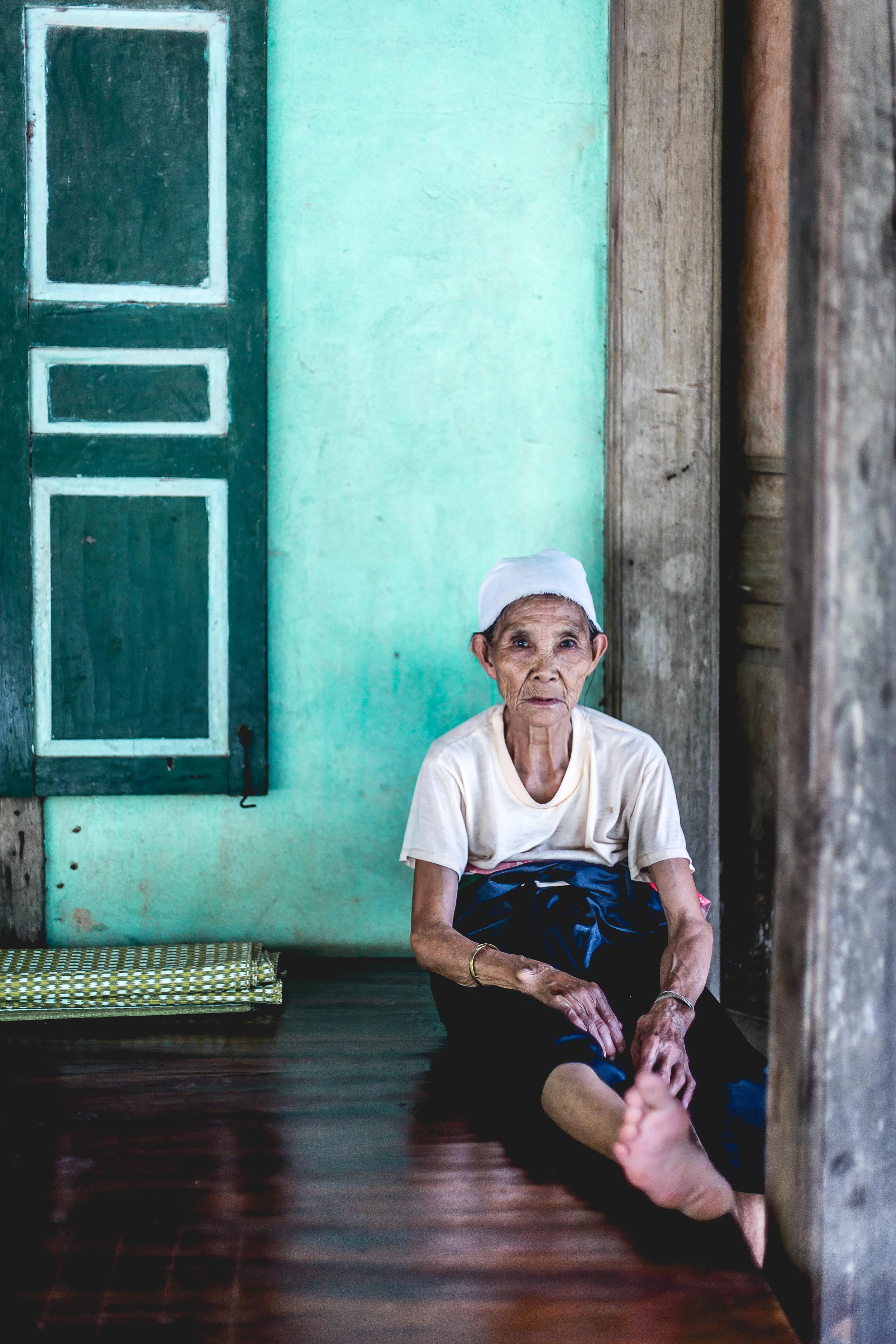
MEDIA COVERAGE
Our latest research reinforces the link between diversity and company financial performance—and suggests how organizations can craft better inclusion strategies for a competitive edge.
Awareness of the business case for inclusion and diversity is on the rise. While social justice typically is the initial impetus behind these efforts, companies have increasingly begun to regard inclusion and diversity as a source of competitive advantage, and specifically as a key enabler of growth. Yet progress on diversification initiatives has been slow. And companies are still uncertain about how they can most effectively use diversity and inclusion to support their growth and value-creation goals.
Recent studies indicate that the face recognition technology used in consumer devices can discriminate based on gender and race.
A new study out of the M.I.T Media lab indicates that when certain face recognition products are shown photos of a white man, the software can correctly guess the gender of the person 99 per cent of the time. However, the study found that for subjects with darker skin, the software made more than 35 per cent more mistakes.
As part of the Gender Shades project 1,270 photos were chosen of individuals from three African countries and three European countries and were evaluated with (AI) products from IBM, Microsoft and Face++-. The photos were classified further by gender and by skin colour before testing them on these products.
The study notes that while each company appears to have a relatively high rate of accuracy overall, of between 87 and 94 per cent, there were noticeable differences in the misidentified images in different groups.
Full article:
https://globalnews.ca/news/4019123/facial-recognition-software-work-white-male-report/
Humanity faces a wide range of challenges that are characterised by extreme complexity, from climate change to feeding and providing healthcare for an ever-expanding global population. Left unchecked, these phenomena have the potential to cause devastation on a previously untold scale. Fortunately, developments in AI could play an innovative role in helping us address these problems.
At the same time, the successful integration of AI technologies into our social and economic world creates its own challenges. They could either help overcome economic inequality or they could worsen it if the benefits are not distributed widely. They could shine a light on damaging human biases and help society address them, or entrench patterns of discrimination and perpetuate them. Getting things right requires serious research into the social consequences of AI and the creation of partnerships to ensure it works for the public good.
While lots of people worry about artificial intelligence becoming aware of itself, then running amok and taking over the world, others are using it to uncover gender bias in the workplace. And that’s more than a little ironic, since AI actually injects not just gender, but racial bias into its data—and that has real-world consequences.
A Fox News report highlights the research with AI that reveals workplace bias, uncovered by research from Boston-based Palatine Analytics. The firm, which studies workplace issues, “analyzed a trove of data—including employee feedback and surveys, gender and salary information and one-on-one check-ins between managers and employees—using the power of artificial intelligence.”
In 1998, the incoming freshman class at Yale University was shown a psychological test that claimed to reveal and measure unconscious racism. The implications were intensely personal. Even students who insisted they were egalitarian were found to have unconscious prejudices (or “implicit bias” in psychological lingo) that made them behave in small, but accumulatively significant, discriminatory ways. Mahzarin Banaji, one of the psychologists who designed the test and leader of the discussion with Yale’s freshmen, remembers the tumult it caused. “It was mayhem,” she wrote in a recent email to Quartz. “They were confused, they were irritated, they were thoughtful and challenged, and they formed groups to discuss it.”
Finally, psychologists had found a way to crack open people’s unconscious, racist minds. This apparently incredible insight has taken the test in question, the Implicit Association Test (IAT), from Yale’s freshmen to millions of people worldwide. Referencing the role of implicit bias in perpetuating the gender pay gap or racist police shootings is widely considered woke, while IAT-focused diversity training is now a litmus test for whether an organization is progressive.
This acclaimed and hugely influential test, though, has repeatedly fallen short of basic scientific standards.
Full article: https://qz.com/1144504/the-world-is-relying-on-a-flawed-psychological-test-to-fight-racism/
The latest fashion trend with most of my clients is Unconscious Bias Training. While those trainings are interesting and engaging, and may raise awareness about various biases, there's little evidence to their effectiveness in eliminating those. This is well explained in Diversity and Inclusion specialist's Lisa Kepinski's article, Unconscious Bias Awareness Training is Hot, But the Outcome is Not: So What to Do About It?
Lisa outlines two problems with these trainings:
- The "So What?" effect: having done the training, leaders and HR professionals alike remain at loss for the next steps that could deliver a sustainable cultural change, and
- The training may backfire by encouraging more biased thinking and behaviors (by conditioning the stereotypes). Moreover, "by hearing that others are biased and it's ‘natural’ to hold stereotypes, we feel less motivated to change biases and stereotypes are strengthened (‘follow the herd’ bias)."
However quickly artificial intelligence evolves, however steadfastly it becomes embedded in our lives -- in health, law enforcement, sex, etc. -- it can't outpace the biases of its creators, humans. Microsoft Researcher Kate Crawford delivered an incredible keynote speech, titled "The Trouble with Bias" at Spain's Neural Information Processing System Conference on Tuesday.
One afternoon in Florida in 2014,18-year Brisha Borden was running to pick up her god-sister from school when she spotted an unlocked kid’s bicycle and a silver scooter. Brisha and a friend grabbed the bike and scooter and tried to ride them down the street. Just as the 18-year-old girls were realizing they were too big for the toys, a woman came running after them saying, “That’s my kid’s stuff.” They immediately dropped the stuff and walked away. But it was too late — a neighbor who witnessed the event had already called the police. Brisha and her friend were arrested and charged with burglary and petty theft for the items, valued at a total of $80.
The previous summer, 41-year-old Vernon Prater was picked up for shoplifting $86.35 worth of tools from a nearby Home Depot store. He had already been convicted of several armed robbery charges and had served 5 years in prison. Borden, the 18 year old, had a record too — but for juvenile misdemeanors.
For the the full transcript and podcast:
https://medium.com/nevertheless-podcast/transcript-garbage-in-garbage-out-78b74b08f16e
Gender equality remains frustratingly elusive. Women are underrepresented in the C-suite, receive lower salaries, and are less likely to receive a critical first promotion to manager than men. Numerous causes have been suggested, but one argument that persists points to differences in men and women’s behavior.
Which raises the question: Do women and men act all that differently? We realized that there’s little to no concrete data on women’s behavior in the office. Previous work has relied on surveys and self-reported assessments — methods of data collecting that are prone to bias. Fortunately, the proliferation of digital communication data and the advancement of sensor technology have enabled us to more precisely measure workplace behavior.
We decided to investigate whether gender differences in behavior drive gender differences in outcomes at one of our client organizations, a large multinational firm, where women were underrepresented in upper management. In this company, women made up roughly 35%–40% of the entry-level workforce but a smaller percentage at each subsequent level. Women made up only 20% of people at the two highest seniority levels at this organization.
Western, educated, industrialised, rich and democratic (WEIRD) norms are distorting the cultural perspective of new technologies
From what we see in our internet search results to deciding how we manage our investments, travel routes and love lives, algorithms have become a ubiquitous part of our society. Algorithms are not just an online phenomenon: they are having an ever-increasing impact on the real-world. Children are being born to couples who were matched by dating site algorithms, whilst the navigation systems for driverless cars are poised to transform our roads.
GALLERY
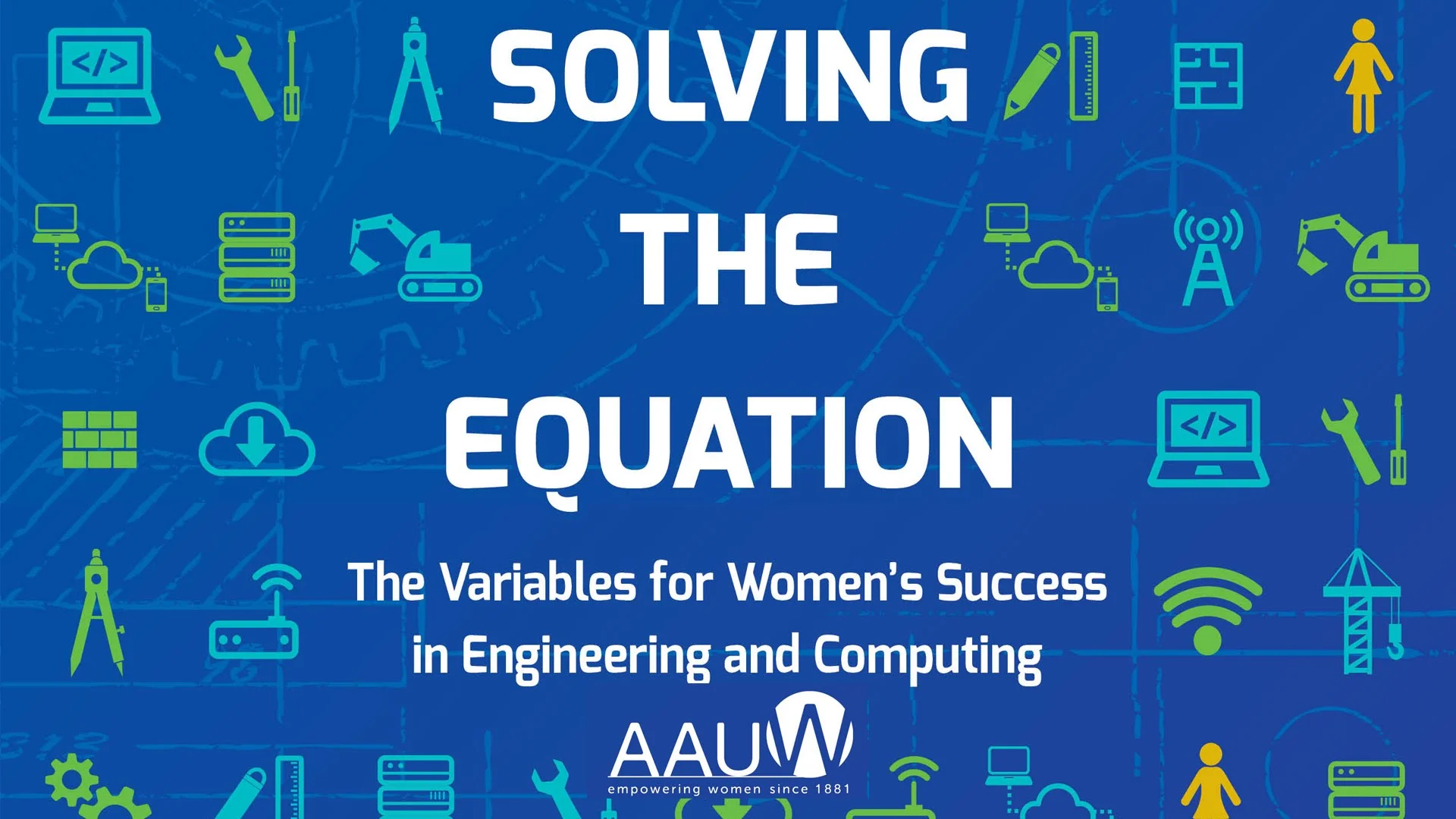
From the research team who brought you Why So Few? Women in Science, Technology, Engineering, and Mathematics comes a deeper dive into engineering and computing, fields that offer 80 percent of STEM jobs but still boast dismal numbers of women students and workers.
Watch report co-authors Catherine Hill, AAUW vice president of research, and Christianne Corbett, AAUW senior researcher and Stanford Professor, discuss Solving the Equation's major findings.
AAUW research.
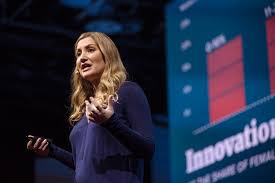
Are diverse companies really more innovative? Rocío Lorenzo and her team surveyed 171 companies to find out - and the answer was a clear yes. In a talk that will help you build a better, more robust company, Lorenzo dives into the data and explains how your company can start producing fresher, more creative ideas by treating diversity as a competitive advantage.

- Géraldine Amiel, Paris Bureau Chief, Bloomberg News
- Bernise Ang, Founder and Executive Director, Zeroth Labs
- Patrice Caine, CEO, Thales
- Melissa Cefkin, Principal Scientist, Design Anthropologist, Renault-Nissan
- Mitsubishi Alliance
- Kimberly Lein-Mathisen, General Manager, Microsoft Norway
Anita Sarkeesian decided to use Feminist Frequency, her video series on the portrayal of women in the media, to document sexist stereotypes and cliches in videogames. That project, Tropes vs. Women in Videogames, ignited a sustained campaign of violent threats and abuse, while raising over $150,000 from nearly 7,000 supporters. Despite the intense scrutiny, each video is essential viewing, a free masterclass in gender studies.
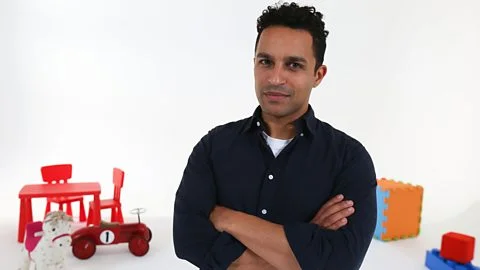
Is the way we treat boys and girls the real reason we haven't achieved equality between men and women? Dr Javid Abdelmoneim aims to find out by taking over a primary school class.
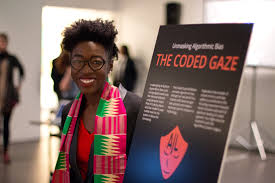
MIT grad student Joy Buolamwini was working with facial analysis software when she noticed a problem: the software didn't detect her face — because the people who coded the algorithm hadn't taught it to identify a broad range of skin tones and facial structures. Now she's on a mission to fight bias in machine learning, a phenomenon she calls the "coded gaze." It's an eye-opening talk about the need for accountability in coding ... as algorithms take over more and more aspects of our lives.

The Chasing Grace Project is a documentary series about women in tech. It includes six episodes, each focused on a different topic within the women in tech narrative. From the pay gap, online harassment and female entrepreneurship to access to the best jobs, the decision to leave or stay in tech and the role of male allies, the series illustrate how we pave the way forward. Through story we can call out the adversities women face and illustrate how they’re navigating their own paths. The result? A series of blueprints for other women to find their paths, their way.
Learn more at:
chasinggracefilm.com
ACADEMIC RESEARCH QUOTED
- Aaron C. Kay
- Adam Kalai
- AI
- algorithms
- Anupam Datta
- Berkman Klein Center
- Black Box
- Classification
- Computation and Language
- Computer Vision
- Cornell University
- Design
- Explanation
- Films
- GloVe
- Gogle.org
- Innovation Group
- James Zou
- Kai-Wei Chang
- Maarten Sap
- machine learning
- Matthias Spielkamp
- Michal Kosinski
- MIT Media Lab
- Nathan Srebro
- Nicholas Diakopoulos
- Privacy
- Signal Analysis and Interpretation Laboratory
- Solon Barocas
- Stanford University
- Talent Management
- The Royal Society
- University of Melbourne
- University of Pennsylvania
- Venkatesh Saligrama
Media Coverage QUOTED
- Academic
- AI
- Alexa
- algorithms
- Bias
- BIAS
- Cathy O'Neil
- Conceptnet
- Cortana
- Fortune
- Guardian
- Havard Kennedy School
- HBR
- Hiring
- Inequality
- Joanna Bryson
- Kate Crawford
- Luminoso
- Machine Learning
- McKinsey
- Microsoft
- ProPublica
- Robots
- Uncounscious Bias
- University of Bath
- University of Washington
- Washington Post
- WEF
- Wired
- Women in Data Science
- World Economic Forum
ELPHA LOVES
This guide was written with the intention of empowering women to navigate the internet without fear. We discuss common occurrences in which women are subject to harassment in their daily lives – on social media, at work, while dating, and more – and give tips and advice on how women can take control.
First Sector-Neutral Bloomberg Gender-Equality Index
Over 100 companies from ten sectors headquartered in 24 countries and regions joined the inaugural 2018 Bloomberg Gender-Equality Index (GEI). The reference index measures gender equality across internal company statistics, employee policies, external community support and engagement, and gender-conscious product offerings.
The Unconscious Bias Project is a group of scientists, tech workers, and artists working together to promote diversity in Science, Technology, Engineering and Mathematics (STEM) fields.
Character portrayal analyses using computational tools
Quick links: [paper] [download the connotation frames] [contact us]
How a movie character is written or portrayed influences a viewer's impression, which can in turn influence people's stereotypes on gender norms. We develop a computational framework, called connotation frames, to measure the power and agency given to characters in movies. Our new tool allows for in-depth analyses of subtle nuances in how characters are written about in movie screenplays.
The Equality Act 2010 (Gender Pay Gap Information) Regulations 2017 apply to private and voluntary-sector organisations with 250 or more employees requiring employers to publish data on their gender pay gaps came into effect on 6th April 2017.
Cindy Gallop, a former BBH chair, always has advice for women making their way in the ad industry. Now she's also giving advice as a chatbot. R/GA partnered with The Muse, Ladies Get Paid, Reply.ai and PayScale to launch "Ask For a Raise" on Equal Pay Day. To access the bot on Facebook Messenger, users can search @AskCindyGallop and message away.
The team used machine-learning-based tools to analyze the language in nearly 800 movie scripts, quantifying how much power and agency those scripts give to individual characters. In their study, recently presented in Denmark at the 2017 Conference on Empirical Methods in Natural Language Processing, the researchers found subtle but widespread gender bias in the way male and female characters are portrayed.
The Select Committee on Artificial Intelligence was appointed on 29 June 2017 to consider the economic, ethical and social implications of advances in artificial intelligence, and to make recommendations.
- Liaison Committee report: New investigative committees in the 2017-18 Session (PDF)
- Liaison Committee report: New investigative committees in the 2017-18 Session (HTML)
The Committee was established following the recommendation of the Liaison Committee. It will report by 31 March 2018.
Many factors affect how much you are paid, including the sector you work in, your age and how long you have been in a job.
But thanks to a law forcing UK employers to publish details of the differences between male and female pay and bonuses for the first time, there is a new spotlight on how gender affects pay.
The average woman working full-time in the UK earns 9.1 per cent less than a man per hour. With part-timers included, the gap is 18.4 per cent.
Find out whether there is a gender pay gap for your job and, if so, what size it is, with our calculator below.
The calculator only has data for full-time workers.
The IEEE Global Initiative for Ethical Considerations in Artificial Intelligence and Autonomous Systems
An incubation space for new standards and solutions, certifications and codes of conduct,
and consensus building for ethical implementation of intelligent technologies.
The IEEE mission is to ensure every stakeholder involved in the design and development of autonomous and intelligent systems is educated, trained, and empowered to prioritize ethical considerations so that these technologies are advanced for the benefit of humanity.
The AI Now Institute at New York University is an interdisciplinary research institute dedicated to understanding the social implications of artificial intelligence. Its work focuses on four core domains: labor and automation, bias and inclusion, rights and liberties, and safety and critical infrastructure.
The AI Now Institute has officially launched in November of 2017. You can learn more at www.ainowinstitute.org
2017 EDITION OF THE GLOBAL GENDER GAP REPORT.
The Global Gender Gap Report benchmarks 144 countries on their progress towards gender parity across four thematic dimensions: Economic Participation and Opportunity, Educational Attainment, Health and Survival, and Political Empowerment. In addition, 2017’s edition also analyses the dynamics of gender gaps across industry talent pools and occupations.
https://www.weforum.org/reports/the-global-gender-gap-report-2017
The median salary for women working full-time is about 80 percent of men’s. That gap, put in other terms, means women are working for free 10 weeks a year. So, if you’re a woman ...... you started working for free 15 hours ago...
Well, that is a little blunt — there are gradients on that difference. The pay gap varies depending on the occupation, working hours, education attainment, experience, and geography.
Look at when women in varying occupations would start working for free, based on the wage gap in that field (US data).
Published 26/10/17
The F-Rating is applied to films by cinemas and film festivals giving moviegoers an easily identifiable label so they can choose films that fairly represent women on screen and behind the camera. Highlighting these films sends a clear message to distributors, producers and funders that women can and should have more than just a supporting role within the industry.
The F-Rating is applied to all films which are directed by women and/or written by women. If the film ALSO has significant women on screen, it receives a TRIPLE F-Rating, our gold standard. The rating allows audiences to “vote with your seat” and proactively choose to go and see F-Rated films.
Through the Global Gender Gap Report, the World Economic Forum quantifies the magnitude of gender disparities and tracks their progress over time, with a specific focus on the relative gaps between women and men across four key areas: health, education, economy and politics. The 2016 Report covers 144 countries. More than a decade of data has revealed that progress is still too slow for realizing the full potential of one half of humanity within our lifetimes.
Gender Pay Gap legislation (developed by the Government Equalities Office) introduced in April 2017 requires all employers of 250 or more employees to publish their gender pay gap for workers in scope as of 31 March 2017.
The intervention is called the Feminist Chatbot Design Process (FCDP), which is a series of reflective questions incorporating feminist interaction design characteristics, ethical AI principles and research on debiasing data. The FCDP encourages design and development teams to follow the reflective questions at the conceptual design phase, and can be used by all team members (technical and non-technical). The outcome of the FCDP is that teams produce a chatbot design which is sensitive to feminist critiques of technology and AI, and grow their own awareness of the relationship between gender power relations and technology.
Created by: Josie Swords | jswor002@gold.ac.uk | 07479 859 470 | @swordstoyoung
FHI is a multidisciplinary research institute at the University of Oxford. Academics at FHI bring the tools of mathematics, philosophy, social sciences, and science to bear on big-picture questions about humanity and its prospects. The Institute is led by founding Director Professor Nick Bostrom.
Humanity has the potential for a long and flourishing future. Our mission is to shed light on crucial considerations that might shape our future.
We designed the Partnership on AI, in part, so that we can invest more attention and effort on harnessing AI to contribute to solutions for some of humanity’s most challenging problems, including making advances in health and wellbeing, transportation, education, and the sciences.
PAIR is devoted to advancing the research and design of people-centric AI systems. We're interested in the full spectrum of human interaction with machine intelligence, from supporting engineers to understanding everyday experiences with AI.
We believe our digital society – the ways we live and work together in the 21st century – should be just as important as our digital economy. That’s why we’re fighting for a fairer internet: one we can all understand and help shape for the future.
The Women’s Business Council is a government-backed, business-led council that was established in 2012 with the aim of ensuring real action to maximise women’s contribution to economic growth.
The Chasing Grace Project is a documentary series about women in tech. It includes six episodes, each focused on a different topic within the women in tech narrative. From the pay gap, online harassment and female entrepreneurship to access to the best jobs, the decision to leave or stay in tech and the role of male allies, the series illustrate how we pave the way forward. Through story we can call out the adversities women face and illustrate how they’re navigating their own paths. The result? A series of blueprints for other women to find their paths, their way.
The code used in the film analysis "She Giggles, He Gallops" is publicly available on GitHub. The data set for this analysis included 1,966 scripts for films released between 1929 and 2015; most are from 1990 and after. Each script was processed to extract only the screen directions, excluding dialogue from this analysis. We then identified all bigrams in these scripts that had either “he” or “she” as the first word in the bigram.
Textio’s predictive engine is fueled by global hiring data. More than 10 million new job posts and their real-world outcomes are added every month. Textio analyzes this data to find the meaningful language patterns that cause some posts to succeed where others fail. Then it feeds that guidance back to users, creating a learning loop that gets smarter with every keystroke.
Textio offers a free scoring tool for companies : https://textio.com/
Our mission at the Leverhulme Centre for the Future of Intelligence (CFI) is to build a new interdisciplinary community of researchers, with strong links to technologists and the policy world, and a clear practical goal: to work together to ensure that we humans make the best of the opportunities of artificial intelligence as it develops over coming decades.
The Bechdel Test, sometimes called the Mo Movie Measure or Bechdel Rule is a simple test which names the following three criteria: (1) it has to have at least two women in it, who (2) who talk to each other, about (3) something besides a man. The test was popularized by Alison Bechdel's comic Dykes to Watch Out For, in a 1985 strip called The Rule. For a nice video introduction to the subject please check out The Bechdel Test for Women in Movies on feministfrequency.com.
Project Implicit is a non-profit organization and international collaboration between researchers who are interested in implicit social cognition - thoughts and feelings outside of conscious awareness and control. The goal of the organization is to educate the public about hidden biases and to provide a “virtual laboratory” for collecting data on the Internet.
2 tests are specifically relevant to gender: Gender Career Test and Gender Science Test
Go to : https://implicit.harvard.edu/implicit/takeatest.html
Project Include’s mission is to give everyone a fair chance to succeed in tech. We are a non-profit that uses data and advocacy to accelerate diversity and inclusion solutions in the tech industry.