More Evidence
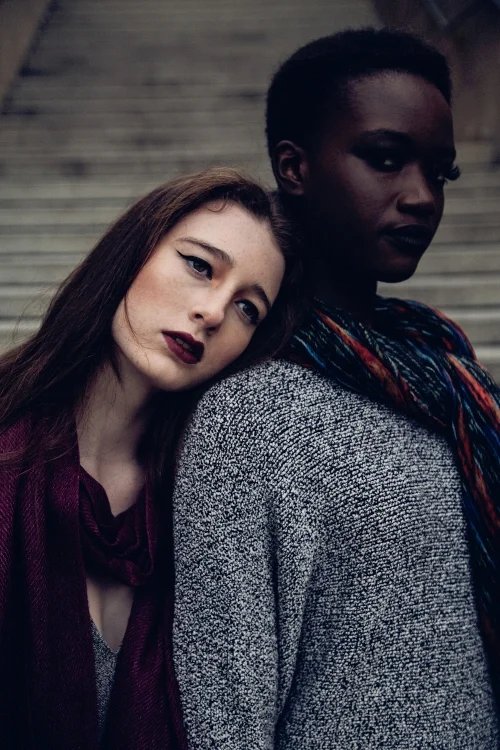
If not careful, AI could entrench gender bias. .
Recent studies indicate that the face recognition technology used in consumer devices can discriminate based on gender and race.
A new study out of the M.I.T Media lab indicates that when certain face recognition products are shown photos of a white man, the software can correctly guess the gender of the person 99 per cent of the time. However, the study found that for subjects with darker skin, the software made more than 35 per cent more mistakes.
As part of the Gender Shades project 1,270 photos were chosen of individuals from three African countries and three European countries and were evaluated with (AI) products from IBM, Microsoft and Face++-. The photos were classified further by gender and by skin colour before testing them on these products.
The study notes that while each company appears to have a relatively high rate of accuracy overall, of between 87 and 94 per cent, there were noticeable differences in the misidentified images in different groups.
Full article:
https://globalnews.ca/news/4019123/facial-recognition-software-work-white-male-report/
Vice-présidente Bots et intelligence artificielle chez Sage, Kriti Sharma est une pionnière dans le développement de machines intelligentes capables de fonctionner et de réagir comme des êtres humains pour simplifier les tâches administratives des entreprises. Elle est aussi la créatrice de Pegg, le premier chatbot de comptabilité au monde qui sera sera commercialisé en 2018 en France et désormais adopté dans 135 pays.
L’intelligence artificielle est une des plus grandes révolutions de notre temps pouvant mettre en danger le pouvoir de l’être humain et son travail. Quel est votre point de vue ?
Kriti Sharma: L’intelligence artificielle est comme n’importe quelle autre révolution technologique majeure, elle aura des implications positives comme négatives. Maintenant, il faut être sûr qu’elles sont utilisées à de bonnes fins. Par exemple pour les petites entreprises qui n’ont pas beaucoup d’équipes technologiques, l’intelligence artificielle peut les aider à automatiser un certain nombre de process.
Par ailleurs, la technologie attire une main d’œuvre de plus en plus diversifiée, ce qui n’existait pas auparavant. L’intelligence artificielle peut également s’automatiser elle-même. Avant, créer un software prenait du temps, maintenant, l’IA commence à écrire ses propres codes. Elle peut, dans une certaine mesure, automatiser le travail de l’ingénieur software. Donc nous avons maintenant un besoin de gens aux compétences créatives, plus seulement des ingénieurs mais une combinaison de profils Art et Science. Autrement dit, vous n’avez pas besoin d’être un ingénieur ou un Data scientifique avec un master pour travailler dans l’intelligence artificielle.
Dans « the end of the professions » David Susskind évoque des professions comme les avocats, qui vont être impactées par l’automatisation et l’intelligence artificielle. Ne pensez-vous pas que cela va accroitre les inégalités à l’échelle mondiale ?
Humanity faces a wide range of challenges that are characterised by extreme complexity, from climate change to feeding and providing healthcare for an ever-expanding global population. Left unchecked, these phenomena have the potential to cause devastation on a previously untold scale. Fortunately, developments in AI could play an innovative role in helping us address these problems.
At the same time, the successful integration of AI technologies into our social and economic world creates its own challenges. They could either help overcome economic inequality or they could worsen it if the benefits are not distributed widely. They could shine a light on damaging human biases and help society address them, or entrench patterns of discrimination and perpetuate them. Getting things right requires serious research into the social consequences of AI and the creation of partnerships to ensure it works for the public good.
L’être humain est-il menacé par la technologie ? La machine risque-t-elle de le dominer ? Notre dossier spécial pour faire le tri entre fantasmes et réalité.
L’intelligence artificielle (IA) est à la mode. Rien que dans Le Monde et sur Lemonde.fr, le sujet a été évoqué dans 200 articles en 2017, soit presque 15 % de plus qu’en 2016. Il en a été question dans tous les domaines : en économie, en science, et même en politique, puisque le premier ministre, Edouard Philippe, a confié une mission sur la question au député (LRM) mathématicien Cédric Villani, dont les conclusions sont attendues en janvier.
Il reste à savoir ce que cache ce terme. Bien sûr, il y a ces fantastiques percées montrant que des machines surpassent désormais l’homme dans des tâches spécifiques. Dans le secteur de la santé, elles repèrent mieux que les médecins des mélanomes ou des tumeurs du sein sur des images médicales. Dans le transport, elles causent moins d’accidents que des chauffeurs. Sans compter les autres avancées : la reconnaissance vocale, l’art du jeu (poker, go), l’écriture, la peinture ou la musique. En coulisse de ce monde si particulier s’activent les géants du numérique (Google, Facebook, Amazon, Microsoft, IBM, Baidu…) ou des start-up désireuses de leur voler la vedette.
While lots of people worry about artificial intelligence becoming aware of itself, then running amok and taking over the world, others are using it to uncover gender bias in the workplace. And that’s more than a little ironic, since AI actually injects not just gender, but racial bias into its data—and that has real-world consequences.
A Fox News report highlights the research with AI that reveals workplace bias, uncovered by research from Boston-based Palatine Analytics. The firm, which studies workplace issues, “analyzed a trove of data—including employee feedback and surveys, gender and salary information and one-on-one check-ins between managers and employees—using the power of artificial intelligence.”
In 1998, the incoming freshman class at Yale University was shown a psychological test that claimed to reveal and measure unconscious racism. The implications were intensely personal. Even students who insisted they were egalitarian were found to have unconscious prejudices (or “implicit bias” in psychological lingo) that made them behave in small, but accumulatively significant, discriminatory ways. Mahzarin Banaji, one of the psychologists who designed the test and leader of the discussion with Yale’s freshmen, remembers the tumult it caused. “It was mayhem,” she wrote in a recent email to Quartz. “They were confused, they were irritated, they were thoughtful and challenged, and they formed groups to discuss it.”
Finally, psychologists had found a way to crack open people’s unconscious, racist minds. This apparently incredible insight has taken the test in question, the Implicit Association Test (IAT), from Yale’s freshmen to millions of people worldwide. Referencing the role of implicit bias in perpetuating the gender pay gap or racist police shootings is widely considered woke, while IAT-focused diversity training is now a litmus test for whether an organization is progressive.
This acclaimed and hugely influential test, though, has repeatedly fallen short of basic scientific standards.
Full article: https://qz.com/1144504/the-world-is-relying-on-a-flawed-psychological-test-to-fight-racism/
Abstract
As algorithmic decision-making has become synonymous with inexplicable decision-making, we have become obsessed with opening the black box. This Article responds to a growing chorus of legal scholars and policymakers demanding explainable machines. Their instinct makes sense; what is unexplainable is usually unaccountable. But the calls for explanation are a reaction to two distinct but often conflated properties of machine-learning models: inscrutability and non intuitiveness. Inscrutability makes one unable to fully grasp the model, while non intuitiveness means one cannot understand why the model’s rules are what they are. Solving inscrutability alone will not resolve law and policy concerns; accountability relates not merely to how models work, but whether they are justified.
In this Article, we first explain what makes models inscrutable as a technical matter. We then explore two important examples of existing regulation-by-explanation and techniques within machine learning for explaining inscrutable decisions. We show that while these techniques might allow machine learning to comply with existing laws, compliance will rarely be enough to assess whether decision-making rests on a justifiable basis.
We argue that calls for explainable machines have failed to recognize the connection between intuition and evaluation and the limitations of such an approach. A belief in the value of explanation for justification assumes that if only a model is explained, problems will reveal themselves intuitively. Machine learning, however, can uncover relationships that are both non-intuitive and legitimate, frustrating this mode of normative assessment. If justification requires understanding why the model’s rules are what they are, we should seek explanations of the process behind a model’s development and use, not just explanations of the model itself. This Article illuminates the explanation-intuition dynamic and offers documentation as an alternative approach to evaluating machine learning models.
Full abstract and research here:
http://blog.experientia.com/paper-intuitive-appeal-explainable-machines/
Men and women score similarly in most areas of mathematics, but a gap favoring men is consistently found at the high end of performance. One explanation for this gap, stereotype threat, was first proposed by Spencer, Steele, and Quinn (1999) and has received much attention. We discuss merits and shortcomings of this study and review replication attempts. Only 55% of the articles with experimental designs that could have replicated the original results did so. But half of these were confounded by statistical adjustment of preexisting mathematics exam scores.
Joy Buolamwini joyab@mit.edu MIT Media Lab 75 Amherst St. Cambridge, MA 02139
Timnit Gebru timnit.gebru@microsoft.com Microsoft Research 641 Avenue of the Americas, New York, NY 10011
Recent studies demonstrate that machine learning algorithms can discriminate based on classes like race and gender. In this work, we present an approach to evaluate bias present in automated facial analysis algorithms and datasets with respect to phenotypic subgroups. Using the dermatologist approved Fitzpatrick Skin Type classification system, we characterize the gender and skin type distribution of two facial analysis benchmarks, IJB-A and Adience. We find that these datasets are overwhelmingly composed of lighter-skinned subjects (79.6% for IJB-A and 86.2% for Adience) and introduce a new facial analysis dataset which is balanced by gender and skin type. We evaluate 3 commercial gender classification systems using our dataset and show that darker-skinned females are the most misclassified group (with error rates of up to 34.7%). The maximum error rate for lighter-skinned males is 0.8%. The substantial disparities in the accuracy of classifying darker females, lighter females, darker males, and lighter males in gender classification systems require urgent attention if commercial companies are to build genuinely fair, transparent and accountable facial analysis algorithms.
Keywords: Computer Vision, Algorithmic Audit, Gender Classification
Full Research: http://proceedings.mlr.press/v81/buolamwini18a/buolamwini18a.pdf
The framing of an action influences how we perceive its actor. We introduce connotation frames of power and agency, a pragmatic formalism organized using frame semantic representations, to model how different levels of power and agency are implicitly projected on actors through their actions. We use the new power and agency frames to measure the subtle, but prevalent, gender bias in the portrayal of modern film characters and provide insights that deviate from the well-known Bechdel test. Our contributions include an extended lexicon of connotation frames along with a web interface that provides a comprehensive analysis through the lens of connotation frames.
Introduction As the use and impact of autonomous and intelligent systems (A/IS) become pervasive, we need to establish societal and policy guidelines in order for such systems to remain human-centric, serving humanity’s values and ethical principles. These systems have to behave in a way that is beneficial to people beyond reaching functional goals and addressing technical problems. This will allow for an elevated level of trust between people and technology that is needed for its fruitful, pervasive use in our daily lives. To be able to contribute in a positive, non-dogmatic way, we, the techno-scientific communities, need to enhance our self-reflection, we need to have an open and honest debate around our imaginary, our sets of explicit or implicit values, our institutions, symbols and representations. Eudaimonia, as elucidated by Aristotle, is a practice that defines human well-being as the highest virtue for a society. Translated roughly as “flourishing,” the benefits of eudaimonia begin by conscious contemplation, where ethical considerations help us define how we wish to live. Whether our ethical practices are Western (Aristotelian, Kantian), Eastern (Shinto, Confucian), African (Ubuntu), or from a different tradition, by creating autonomous and intelligent systems that explicitly honor inalienable human rights and the beneficial values of their users, we can prioritize the increase of human well-being as our metric for progress in the algorithmic age. Measuring and honoring the potential of holistic economic prosperity should become more important than pursuing one-dimensional goals like productivity increase or GDP growth.
To partly address people’s concerns over web tracking, Google has created the Ad Settings webpage to provide information about and some choice over the profiles Google creates on users. We present AdFisher, an automated tool that explores how user behaviors, Google’s ads, and Ad Settings interact. AdFisher can run browser-based experiments and analyze data using machine learning and significance tests. Our tool uses a rigorous experimental design and statistical analysis to ensure the statistical soundness of our results. We use AdFisher to find that the Ad Settings was opaque about some features of a user’s profile, that it does provide some choice on ads, and that these choices can lead to seemingly discriminatory ads. In particular, we found that visiting webpages associated with substance abuse changed the ads shown but not the settings page. We also found that setting the gender to female resulted in getting fewer instances of an ad related to high paying jobs than setting it to male. We cannot determine who caused these findings due to our limited visibility into the ad ecosystem, which includes Google, advertisers, websites, and users. Nevertheless, these results can form the starting point for deeper investigations by either the companies themselves or by regulatory bodies.
Adapted by Professor Uta Frith DBE FBA FMedSci FRS from guidance issued to recruitment panels by the Scottish Government
Introduction
All panels and committees for selection and appointments at The Royal Society should be carried out objectively and professionally.
The Society is committed to making funding or award decisions purely on the basis of the quality of the proposed science and merit of the individual. No funding applicant or nominee for awards, Fellowship, Foreign Membership, election to a post or appointment to a committee should receive less favourable treatment on the grounds of: gender, marital status, sexual orientation, gender re-assignment, race, colour, nationality, ethnicity or national origins, religion or similar philosophical belief, spent criminal conviction, age or disability.
The ubiquity of systems using artificial intelligence or “AI” has brought increasing attention to how those systems should be regulated. The choice of how to regulate AI systems will require care. AI systems have the potential to synthesize large amounts of data, allowing for greater levels of personalization and precision than ever before—applications range from clinical decision support to autonomous driving and predictive policing. That said, our AIs continue to lag in common sense reasoning [McCarthy, 1960], and thus there exist legitimate concerns about the intentional and unintentional negative consequences of AI systems [Bostrom, 2003, Amodei et al., 2016, Sculley et al., 2014].
Decisions based on algorithmic, machine learning models can be unfair, reproducing biases in historical data used to train them. While computational techniques are emerging to address aspects of these concerns through communities such as discrimination-aware data mining (DADM) and fairness, accountability and transparency machine learning (FATML), their practical implementation faces real-world challenges. For legal, institutional or commercial reasons, organisations might not hold the data on sensitive attributes such as gender, ethnicity, sexuality or disability needed to diagnose and mitigate emergent indirect discrimination-by-proxy, such as redlining. Such organisations might also lack the knowledge and capacity to identify and manage fairness issues that are emergent properties of complex sociotechnical systems. This paper presents and discusses three potential approaches to deal with such knowledge and information deficits in the context of fairer machine learning. Trusted third parties could selectively store data necessary for performing discrimination discovery and incorporating fairness constraints into model-building in a privacy-preserving manner. Collaborative online platforms would allow diverse organisations to record, share and access contextual and experiential knowledge to promote fairness in machine learning systems. Finally, unsupervised learning and pedagogically interpretable algorithms might allow fairness hypotheses to be built for further selective testing and exploration.
We show that faces contain much more information about sexual orientation than can be perceived and interpreted by the human brain. We used deep neural networks to extract features from 35,326 facial images. These features were entered into a logistic regression aimed at classifying sexual orientation. Given a single facial image, a classifier could correctly distinguish between gay and heterosexual men in 81% of cases, and in 74% of cases for women. Human judges achieved much lower accuracy: 61% for men and 54% for women. The accuracy of the algorithm increased to 91% and 83%, respectively, given five facial images per person. Facial features employed by the classifier included both fixed (e.g., nose shape) and transient facial features (e.g., grooming style).